
Examining the impacts of sociotechnical systems factors on safety in clinical systems.
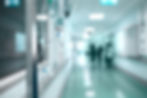
What we do
The Safety, Equity, & Design (SED) lab is directed by Dr. Myrtede Alfred, an assistant professor in the Mechanical and Industrial Engineering at the University of Toronto. The lab examines sociotechnical systems factors contributing to adverse events in clinical systems. Complementing the social determinants of health framework, the research lab also leverages human factors and systems engineering to examine clinical systems’ contributions to racial/ethnic disparities in health outcomes. We are currently conducting AHRQ and NSERC funded research investigating maternal health disparities, remote patient monitoring, and retained foreign objects.
Latest Publications
A Machine Learning Approach with Human-AI Collaboration for Automated Classification of Patient Safety Event Reports: Algorithm Development and Validation Study
Adverse events refer to incidents with potential or actual harm to patients in hospitals. These events are typically documented through patient safety event (PSE) reports, which consist of detailed narratives providing contextual information on the occurrences. Accurate classification of PSE reports is crucial for patient safety monitoring. However, this process faces challenges due to inconsistencies in classifications and the sheer volume of reports. Recent advancements in text representation, particularly contextual text representation derived from transformer-based language models, offer a promising solution for more precise PSE report classification. Integrating the machine learning (ML) classifier necessitates a balance between human expertise and artificial intelligence (AI). Central to this integration is the concept of explainability, which is crucial for building trust and ensuring effective human-AI collaboration. This study aims to investigate the efficacy of ML classifiers trained using contextual text representation in automatically classifying PSE reports. Furthermore, the study presents an interface that integrates the ML classifier with the explainability technique to facilitate human-AI collaboration for PSE report classification.
Applying an equity lens to hospital safety monitoring: a critical interpretive synthesis protocol
Hospital safety monitoring systems are foundational to how adverse events are identified and addressed. They are well positioned to bring equity-related safety issues to the forefront for action. However, there is uncertainty about how they have been, and can be, used to achieve this goal. We will undertake a critical interpretive synthesis (CIS) to examine how equity is integrated into hospital safety monitoring systems. This review will follow CIS principles. Our initial compass question is: How is equity integrated into safety monitoring systems? We will begin with a structured search strategy of hospital safety monitoring systems in CINAHL, EMBASE, MEDLINE and PsycINFO for up to May 2023 to identify papers on safety monitoring systems generally and those linked to equity (eg, racism, social determinants of health). We will also review reference lists of selected papers, contact experts and draw on team expertise. For subsequent literature searching stages, we will use team expertise and expert contacts to purposively search the social science, humanities and health services research literature to support the development of a theoretical understanding of our topic. Following data extraction, we will use interpretive processes to develop themes and a critique of the literature. The above processes of question formulation, article search and selection, data extraction, and critique and synthesis will be iterative and interactive with the goal to develop a theoretical understanding of equity in hospital monitoring systems that will have practice-based implications. This review does not require ethical approval because we are reviewing published literature. We aim to publish findings in a peer-reviewed journal and present at conferences.
Investigating racial and ethnic disparities in maternal care at the system level using patient safety incident reports
Maternal mortality in the United States is high, and women and birthing people of color experience higher rates of mortality and severe maternal morbidity (SMM). More than half of maternal deaths and cases of SMM are considered preventable. Our research investigated systems issues contributing to adverse outcomes and racial/ethnic disparities in maternal care using patient safety incident reports. We reviewed incidents reported in the labor and delivery unit (L&D) and the antepartum and postpartum unit (A&P) of a large academic hospital in 2019 and 2020. Deliveries associated with a reported incident were described by race/ethnicity, age group, method of delivery, and several other process variables. Differences across racial/ethnic group were statistically evaluated. Almost two-thirds (64.8%) of the 528 reports analyzed were reported in L&D, and 35.2% were reported in A&P. Non-Hispanic white (NHW) patients accounted for 43.9% of reported incidents, non-Hispanic Black (NHB) patients accounted for 43.2%, Hispanic patients accounted for 8.9%, and patients categorized as “other” accounted for 4.0%. NHB patients were disproportionally represented in the incident reports as they only accounted for 36.5% of the underlying birthing population. The odds ratio (OR) demonstrated a higher risk of a reported adverse incident for NHB patients; however, adjustment for cesarean section attenuated the association (OR = 1.25; 95% confidence interval = 1.01–1.54). Greater integration of patient safety and health equity efforts in hospitals are needed to promptly identify and alleviate racial and ethnic disparities in maternal health outcomes. While additional systems analysis is necessary, we offer recommendations to support safer, more equitable maternal care.
The problem of algorithmic bias represents an ethical threat to the fair treatment of patients when their care involves machine learning (ML) models informing clinical decision-making. The design, development, testing, and integration of ML models therefore require a lifecycle approach to bias identification and mitigation efforts. Presently, most work focuses on the ML tool alone, neglecting the larger sociotechnical context in which these models operate. Moreover, the narrow focus on technical definitions of fairness must be integrated within the larger context of medical ethics in order to facilitate equitable care with ML. Drawing from principles of medical ethics, research ethics, feminist philosophy of science, and justice-based theories, we describe the Justice, Equity, Fairness, and Anti-Bias (JustEFAB) guideline intended to support the design, testing, validation, and clinical evaluation of ML models with respect to algorithmic fairness. This paper describes JustEFAB's development and vetting through multiple advisory groups and the lifecycle approach to addressing fairness in clinical ML tools. We present an ethical decision-making framework to support design and development, adjudication between ethical values as design choices, silent trial evaluation, and prospective clinical evaluation guided by medical ethics and social justice principles. We provide some preliminary considerations for oversight and safety to support ongoing attention to fairness issues. We envision this guideline as useful to many stakeholders, including ML developers, healthcare decision-makers, research ethics committees, regulators, and other parties who have interest in the fair and judicious use of clinical ML tools.